Undocumented MATLAB
by Octahedron Ltd
Professional MATLAB consulting, development and training
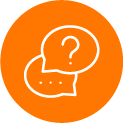
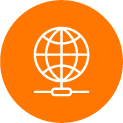
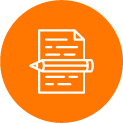
Octahedron Ltd (known as Undocumented Matlab) provides expert MATLAB consulting, development and training since 2009 to organizations and individuals worldwide. The consultancy was founded by Yair Altman, a renowned MATLAB expert with 30 years professional software development experience. Yair is widely recognized as a world-class authority in the MATLAB community and by MathWorks themselves. Yair holds a BSc in Physics and MSc in Computer Science, both with top honors.
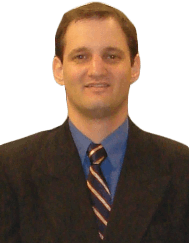
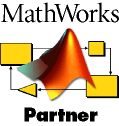
“Yair knows to share his enormous Matlab knowledge in a very efficient and flexible way. The training was structured very well and contained a good and detailed introduction in object oriented Matlab programming. At the same time it contained a huge amount of new ideas and new knowledge for experienced object oriented Matlab programmers. Additionally we got hundreds of ideas to improve the performance, robustness and GUI programming..."
read more
read more
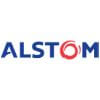
“We brought Yair in for development on our project, and we’re very glad we did. Yair was easy to work with and grasped the work quickly. He demonstrated good software practices, found many little improvements along the way, told us when he would be available to do the work, was done on time and under budget, and even made billing easy. I will be looking to bring him in to more of my projects.”
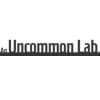
“Yair Altman is a real “Matlab Technologist”. He knows and understands Matlab from the ground up and especially specializes in advanced topics such as Java connectivity, GUI, Matlab performance and Object Oriented.”
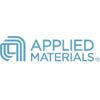
“Yair and his team have assisted us with several complex projects over a number of years, both face to face and remotely. They have been thoroughly professional, efficient, flexible and easy to work with, every time. Yair himself is not only a true guru but also extremely quick and productive in hands-on problem solving. I unreservedly recommend him and his company.”
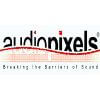
“Yair’s website has been an invaluable source of inspiration and technical information over the years, allowing us to develop much slicker applications in Matlab than we thought possible. I’ve hired him recently to develop a custom UI component, and couldn’t be happier with the professionalism, the respect of deadline, and of course the result.”
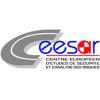
“We hired Yair after two failed attempts with other programming firms. Yair delivered a professional application that is superior to anything else in the market, and cost us a fraction of what the other firms charged. Yair is extremely responsive, often providing fixes or improvements within hours if not days, without unnecessary paperwork or chitchat..."
read more
read more
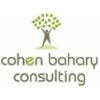
“Working with Yair is a pleasure. He understands immediately the customers needs. He works many orders of magnitude faster than what a less experienced programmer is able to. His knowledge of Matlab greatly exceeds others in depth level, and he extends Matlab based on his knowledge of other languages. He sped up [the application] from practically useless to clinically useful. He communicates with the customer fast and concisely, losing no time in useless chit-chat...”
read more
read more
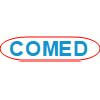
“Yair is extremely knowledgeable, flexible and efficient. I thoroughly recommend Yair as a consultant for advanced MATLAB development and I am looking forward to engaging with him again.”
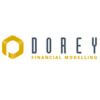
“Octahedron (Yair and his staff) have been a tremendous asset to Flightware in two MATLAB software development projects spanning three years. This was a complex project involving hardware, hardware integration and development of software to perform many functions with a high level of reliability. Not only did Octahedron perform its tasks very well, it did so as one of three MATLAB teams working collaboratively on different tasks across ten time zones...”
read more
read more
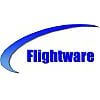
“We had Yair design and build a custom GUI for plotting mixed datasets. Yair worked with us to design the GUI so that it was simpler and easier to use than our original concept. His knowledge of MATLAB and how to construct an intuitive user interface made this project a big success.”
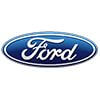
“Excellent work, rapid turnaround. Saved us lots of time and money.”
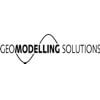
“We brought Yair in as a consultant in the late development stage of a custom MATLAB toolbox. Yair’s proficiency in Java-MATLAB integration saved us a significant amount of time in GUI development. Yair helped us replace several graphics components with Java Swing objects with improved functionality and design. I’d recommend Yair to anyone looking for third-party help with GUI design & deployment out of the MATLAB environment.”
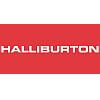
“Yair has a fantastic ability to accelerate Matlab UIs. His deep understanding of Matlab performance has enabled us to work much more efficiently and enjoyably. Cost effective and very fast turn around too. Additionally, Yair is able to see the purpose of a UI and has the experience to see where things can be improved measurably.
The program is now tremendously fast. Just awesome. This is exactly what we were hoping for. This is really making a difference in terms of how fast we can do science!”
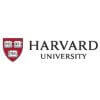
“Yair is on our team of contracting software developers (Matlab, specifically). Yair helped accelerate our work flow, quickly understanding what our software team asked of him; he delivered high quality code; and he demonstrated software practices found in the best coders. We are grateful that he works with us.”
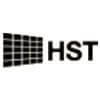
“Yair has been an outstanding resource for us – from speed of execution, to algorithm development, to extending MATLAB beyond its natural borders, his work is consistently thoughtful, thorough, maintainable and prompt. We couldn’t recommend him more highly.”
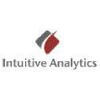
“Yair combines a truly deep and broad knowledge of Matlab, which allow him to single-handedly manage complex projects in an effective manner. He has proved better value for money than the MathWorks consultant available out of Switzerland, and more flexible to work with. Would highly recommend.”
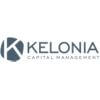
“We brought Yair in for Matlab development projects during the period 2015 to 2017. Yair was highly knowledgeable and easy to work with. He demonstrated good software development practices. I thoroughly recommend Yair as a consultant for MATLAB related projects.”
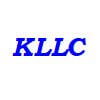
“I had a short consultancy with Yair when he was in Italy. He answered all my questions and showed me code examples and online references to get more information online. I got numerous suggestions on how to prevent errors, improving compiled applications, using java interfaces, and many suggestions on how to improve the quality of my work. After a few questions and answers I simply said: “Yair, this is the software I’m selling, how can I improve it ?” – the audio recording is still on my desktop to this day for reference...”
read more
read more
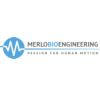
“Yair has been an excellent Matlab consultant. He has delivered consistently great results, efficiently, on time, with minimal training effort. The code he produces not only works well, but the source is well-written with excellent comments. He is also able to design intuitive user interfaces, which can be very challenging for complicated software.”
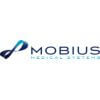
“I was referred to Yair by a trusted colleague for support with integrating a customized datafeed into MATLAB. The complexity was substantial and despite numerous attempts, MathWorks was unable to address the issue. Yair developed an efficient solution and I truly believe he is one of the most skilled MATLAB programmers in the world. I was amazed at the depth of his knowledge from my interactions with him as well as from reading his books. His capabilities have no bounds. He will solve any problem, regardless of the degree of difficulty. It was a pleasure getting to know him.”
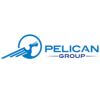
“Yair has helped us in developing our in-house engineering program. His human qualities and contribution was greatly appreciated along with the quality of work, the quick turnaround and the understanding of our needs, I therefore warmly recommend Yair Altman for any challenge you might have with MatLab.”
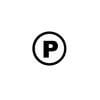
“Yair is the undisputed guru for anything to do with MATLAB’s undocumented features and Java integration. He has helped me many times with seemingly intractable MATLAB GUI issues. His thorough, exhaustively researched and well written blog is must reading for anyone who needs to go beyond the basics when designing powerful, full featured MATLAB user interfaces.”
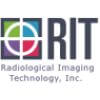
“We hired Yair to write some functions for our prop-shop. Got excellent help within 10 hours and he is eager to solve all the things you need.”
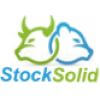
“I worked with Yair both on development projects and tailored Matlab advanced training. On both occasions it has been a great pleasure and great experience. His knowledge of the inner workings of Matlab in general, and Matlab-Java interface is unbelievable. I feel very much enriched from this experience… wonderful!
In the complex project we carried out together, I was truly amazed by how quickly and precisely he grasped all the specifications… and how fast he delivered! I highly recommend his services.”
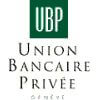
Previous
Next
Speeding-up builtin Matlab functions – part 3
April 6, 2020
Built-in Matlab functions can often be profiled and optimized for improved run-time performance. This article shows a typical example.
Speeding-up builtin Matlab functions – part 3
Built-in Matlab functions can often be profiled and optimized for improved run-time performance. This article shows a typical example.
April 6, 2020
Improving graphics interactivity
April 22, 2019
Matlab R2018b added default axes mouse interactivity at the expense of performance. Luckily, we can speed-up the default axes.
Interesting Matlab puzzle – analysis
April 9, 2019
Solution and analysis of a simple Matlab puzzle that leads to interesting insight on Matlab’s parser.